Drug Discovery: Don’t just add AI
AI has yet to identify effective therapeutics because the ex vivo systems used to train algorithms differ too greatly from the living mammalian systems they are trying to predict.
For AI to have a transformational effect in drug discovery, training data must come from living mammals.
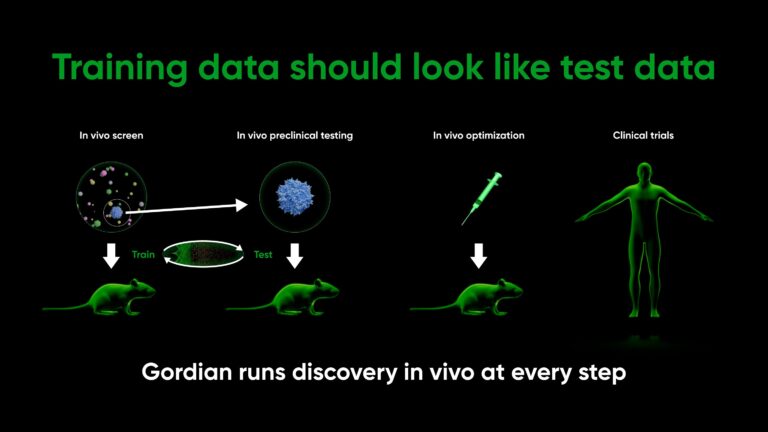
If you’re reading this, you’ve undoubtedly noticed that AI has delivered some incredible advances in the last couple of years. ChatGPT can answer most questions with ease, programmers regularly use tools like Copilot to help them finish code or create it from scratch, fully self-driving cars are operating in several major cities, and anyone can create incredible digital art by typing a few words.
These amazing algorithms have been thrown at basically every industry, including biotechnology. Unfortunately, while success has been impressive in biotechnology outside of drug discovery (AlphaFold, Nobel Prizes, etc), success in telling us how to cure disease has been far from meteoric. Exscientia wound down its Phase I/II study, BenevolentAI’s dermatitis drug failed Phase IIa, and most recently, InSilico and Recursion reported Phase IIa successes but had little to say on efficacy. Recursion lost 16% of its value that day, and InSilico has since scrapped plans for an IPO, again. The headlines paint an uncertain picture at best:
AI tools don’t equal guaranteed drug success as BenevolentAI flops dermatitis test
After years of hype, the first AI-designed drugs fall short in the clinic
With few efficacy details, Insilico claims a Phase 2a win in latest AI readout
Beyond the Hype: Unveiling the Real Impact of Generative AI in Drug Discovery
What gives?
This quote from the last article gives us a clue:
“While generative AI shows promise, the process of transforming an AI-generated idea into a viable therapeutic solution is a challenging task. AI can predict potential drug candidates but validating those candidates through preclinical and clinical trials is where the real challenge begins.”
This points to a fundamental, structural problem: Drug discovery has, and still does, take place in one system, but validates those discoveries in a different system.
Think about it this way. ChatGPT’s output is language. Naturally, it was trained on the compendium of written language. Copilot produces code for programmers and was unsurprisingly trained on code written by programmers. Self-driving cars drive in traffic…and are trained on videos, radar, and lidar gathered from driving in traffic.
Whether a drug cures disease must be validated in a living organism (in vivo). But the need for efficiency has forced drug discovery to seek out simplified systems to run tests at scale*: cultured cells in wells, organoids, explants, tissue slices. AI and robotics have made this process much more efficient, and new ways to measure and perturb systems have been developed, but it is still the same fundamental discovery process. In AI speak, drug discovery models are being trained in the wrong system.
Your training data should look like your test data. You wouldn’t train a self-driving car on the California Vehicle Code. If you’re going to test your drug in a living mammal, you must train for discovery in that living mammal.
*Where discovery has taken place in vivo, the process is expensive, slow, and does not scale.
Redesigning preclinical R&D
Preclinical discovery is complex. Countless articles have described the process, so I will skip the details here, but this image pulled from a quick Google search summarizes the process in 12 (not so) easy steps. 11 of them take place outside of the preclinical animal model system:
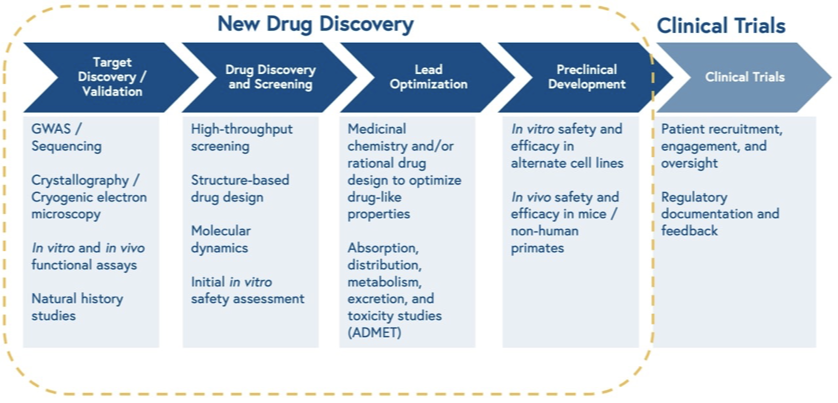
A tell-tale sign that we haven’t taken maximal advantage of the incredible AI, robotics, and omics tools invented recently is that the companies leading this effort have preclinical processes that look essentially the same, just with AI and robotics added. Here is Recursion’s 17 step preclinical process. The in vivo validation animal model comes in on step 17, and reminds us that “validating those candidates through preclinical and clinical trials is where the real challenge begins.”
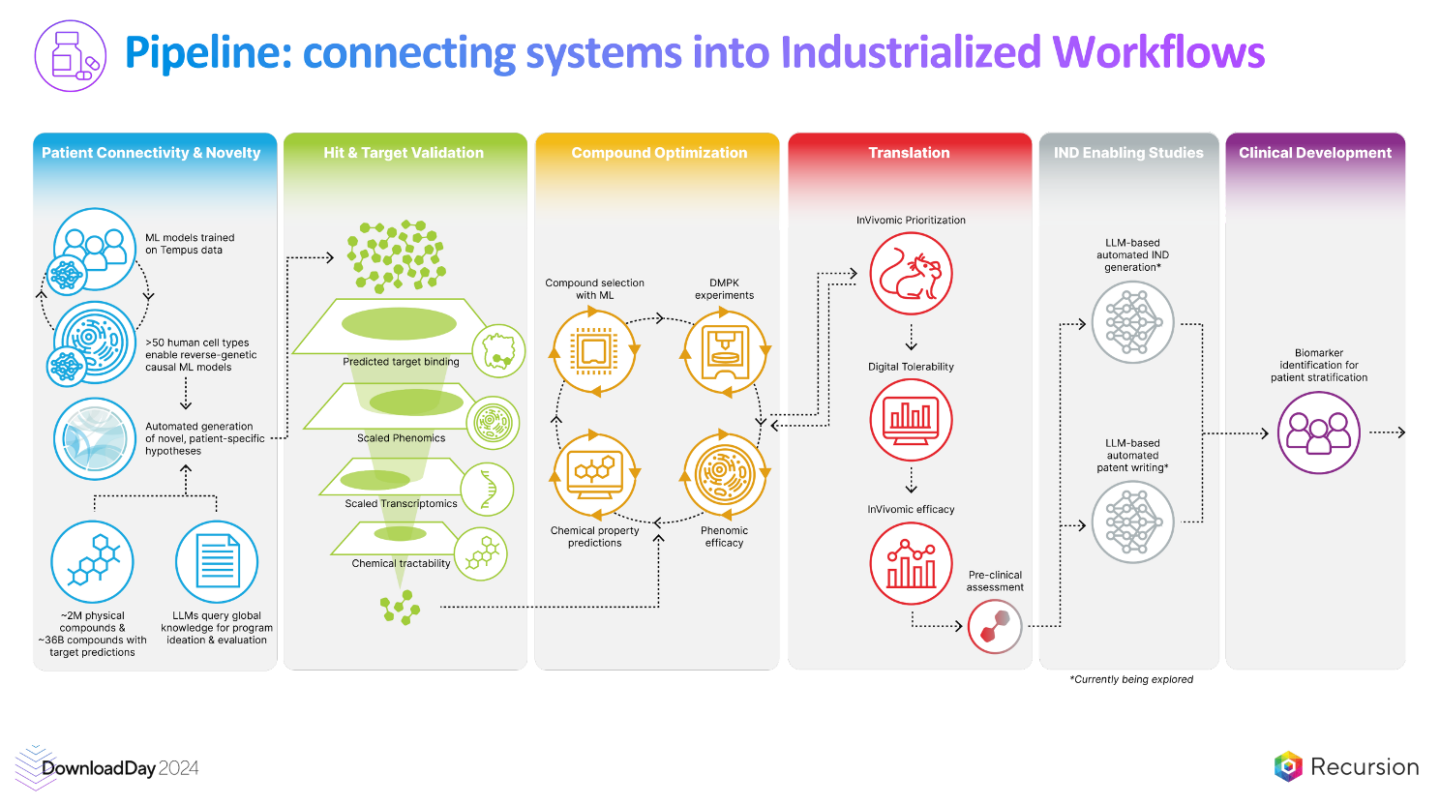
InSilico has 12 steps they’ve transformed with AI. Validation presumably comes before Medicine42:
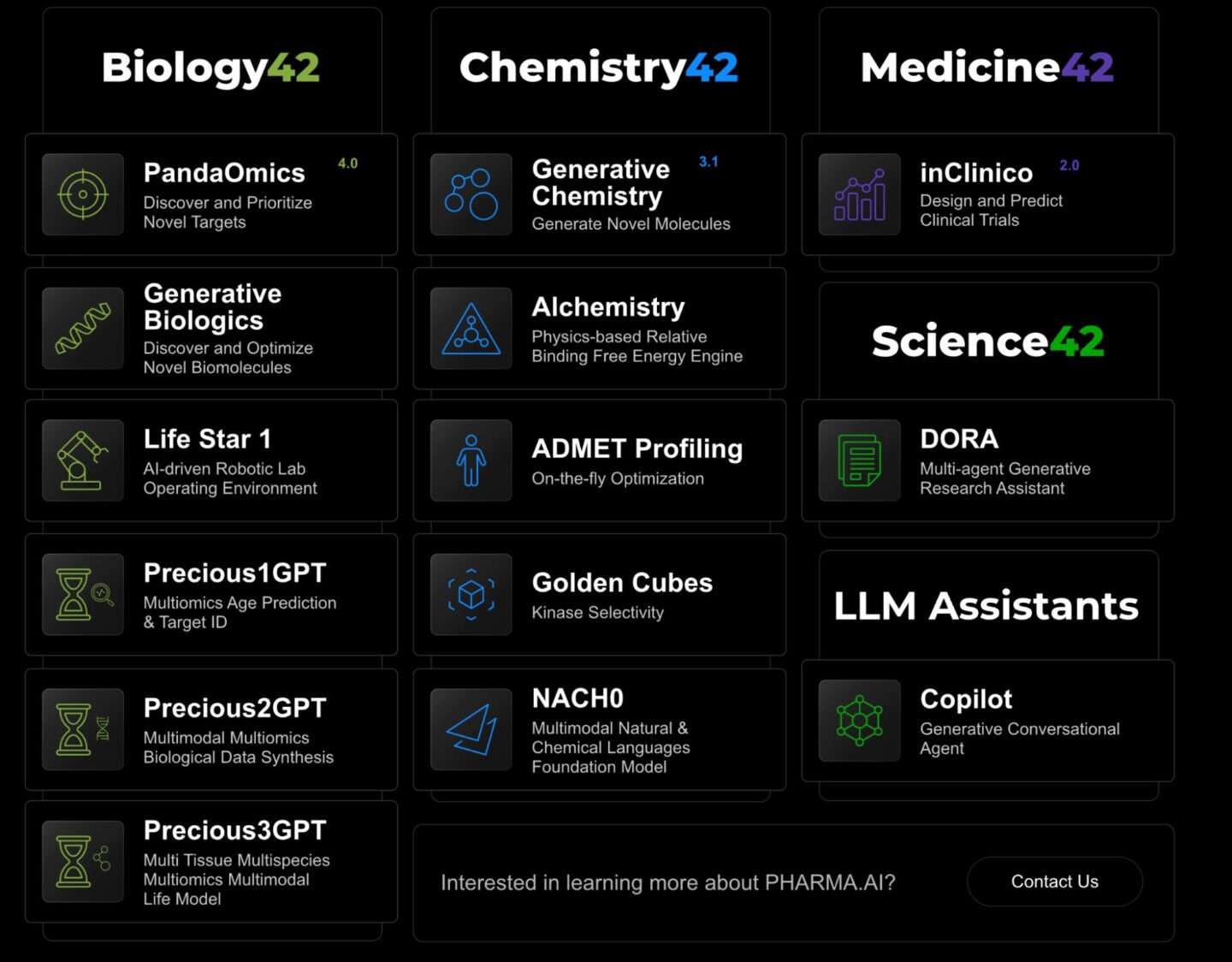
Using AI to make existing processes more efficient is certainly an improvement, but leaves an enormous amount on the table.
A feedback loop built for AI
At Gordian, we’ve redesigned the preclinical process from the ground up. Since all drug developers must validate in animals, Gordian trains for discovery in animals on step one. Our preclinical discovery process takes place entirely in vivo (see The In Vivo Screening Revolution and Introducing High Throughput In Vivo Screening for more detail):
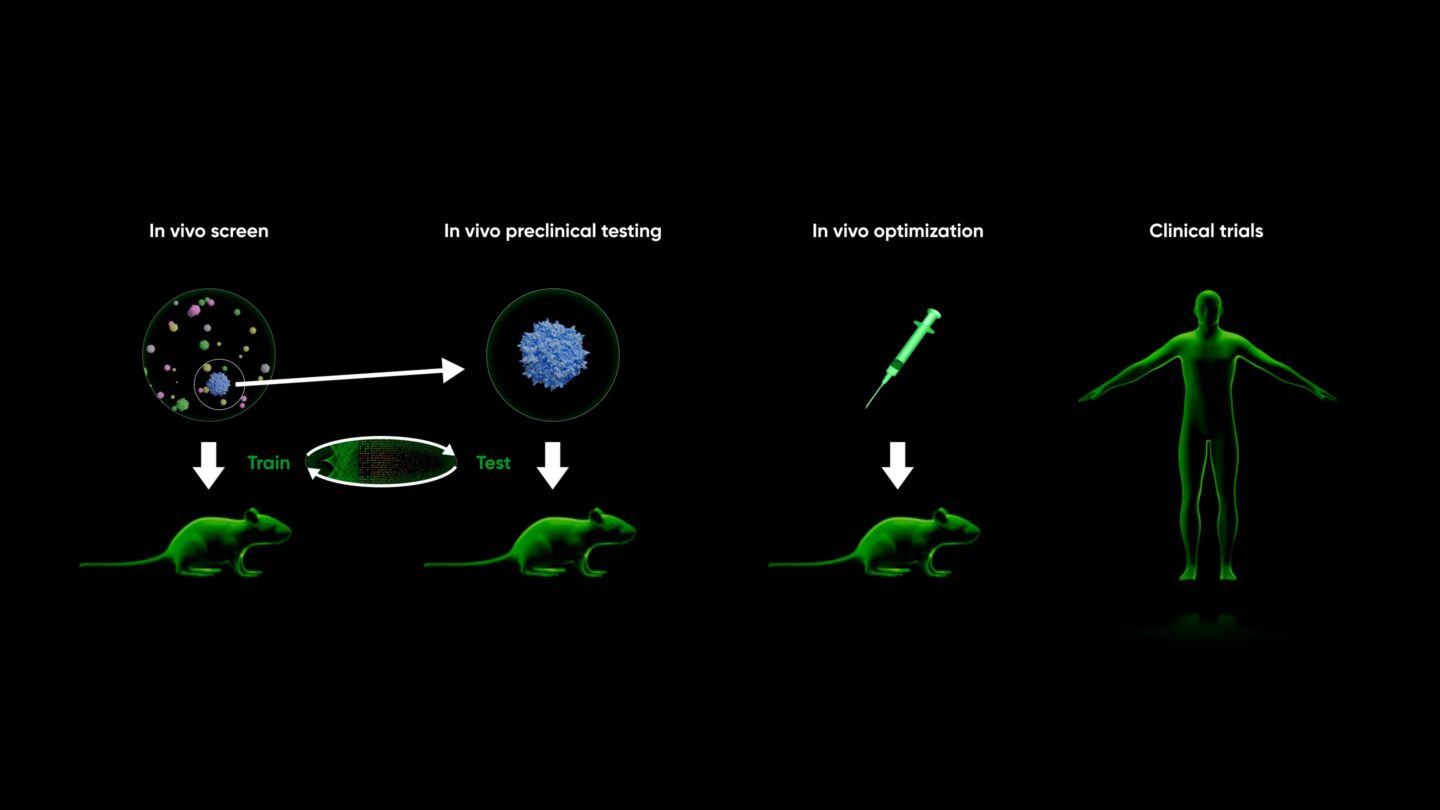
Most importantly, our training data (in vivo screens) comes from the same system as our predict / test data (in vivo preclinical validation), allowing a feedback loop built for AI.
By training and screening in vivo, our predictions for in vivo results are automatically, dramatically better. This isn’t because we use better AI or more advanced assays. This is because training data comes from the same system as our validation system, cutting away the caveats and unknowns of applying conclusions drawn from one system to another.
We can make this training data even more predictive by screening in models that share traits like anatomy and origin of disease. The expense of in vivo experiments lies in the animal model, especially when going beyond young mice. Since we can screen thousands of therapeutics with high confidence, we can bear the cost of much more advanced animal models (we call these Patient Avatars). This directly improves clinical translation:
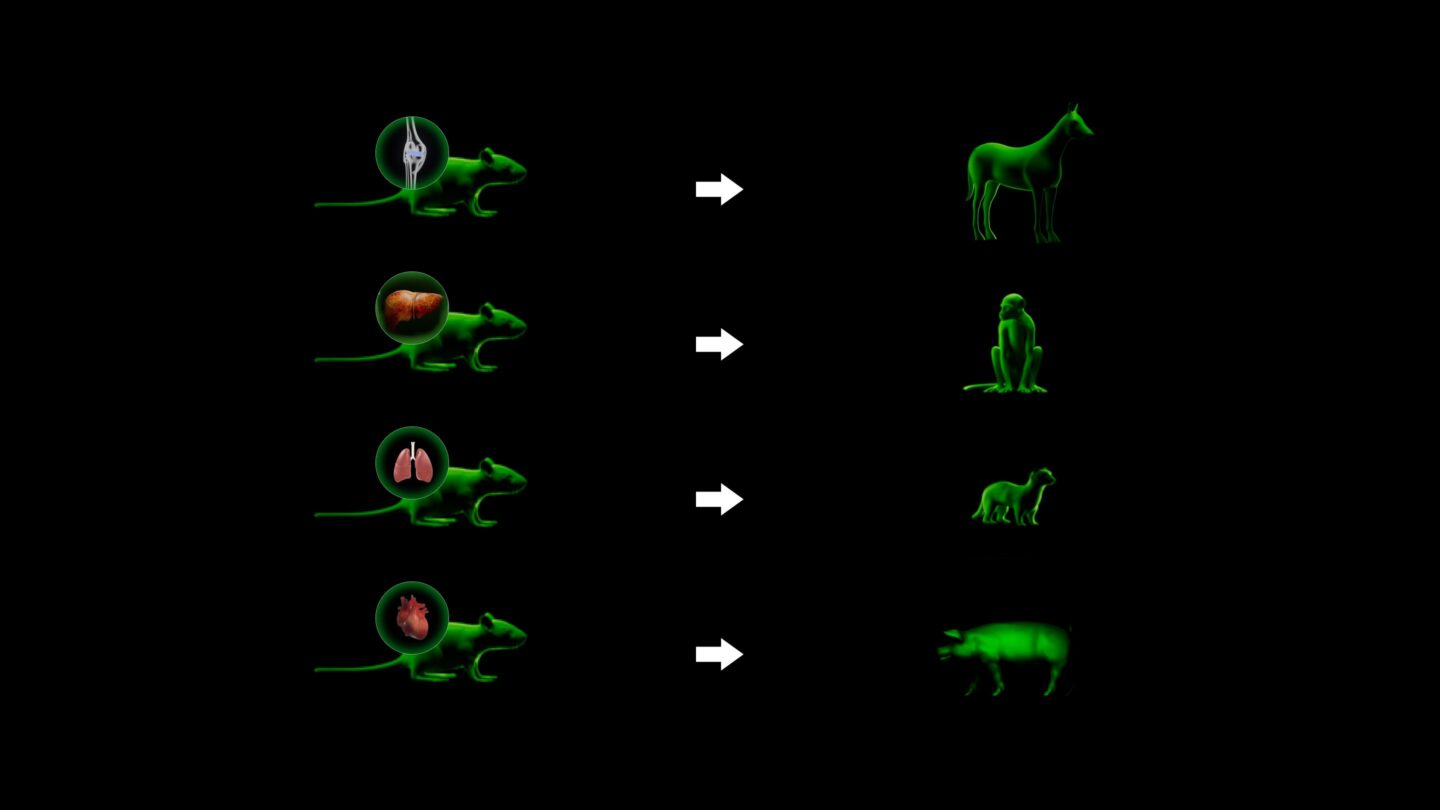
Finally, our platform can produce biologically validated therapeutics at scale. This means that rather than pushing forward a single target/drug that takes millions in sunk cost before assessing whether it looks good enough to advance to trials, we produce dozens of clinically viable therapeutics, rigorously evaluate their potential, and nominate the best for clinical trials.
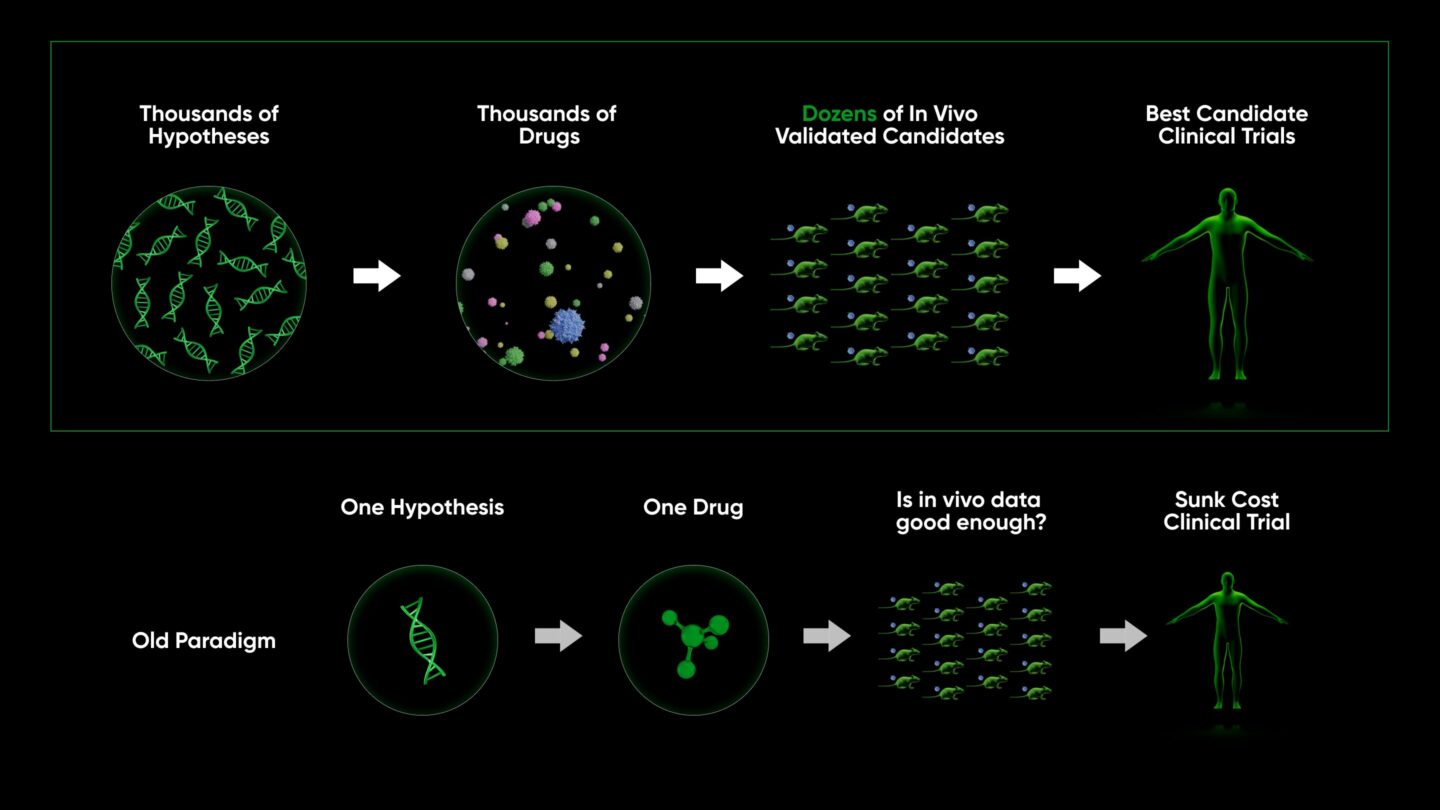
Curing complex, age-related disease
Current applications of AI to drug discovery miss the forest for the trees. AI is used to make the existing drug discovery paradigm more efficient, but the opportunity to correct structural flaws in the legacy discovery process is missed. We believe this has led to the questionable success AI has had in improving drug discovery thus far.
If taken advantage of maximally, existing AI technology has the capacity to radically alter our ability to discover breakthrough drugs, particularly in the complex, age-related disorders that have stymied discovery efforts thus far.